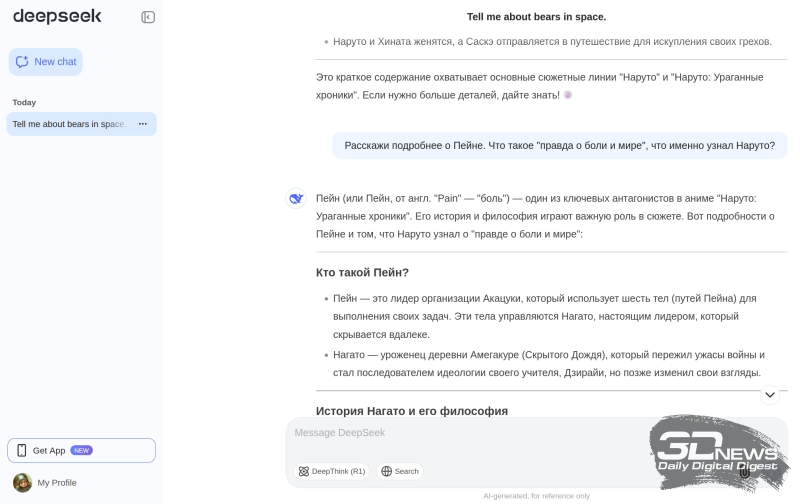
Even Chinese AI models know Naruto is cool (source: DeepSeek screenshot)
⇡#China strikes back
As a number of stock market analysts point out, it was not the very fact of the creation of the DeepSeek R1 generative model in China that caused investors’ nervousness. It had been available via a web interface since December 2024, and its smartphone application had simply blown up the charts by the end of January of this year, taking first place in downloads for iOS in the US, UK, Canada, Singapore and China. The main thing that scared stock market players was the amazingly low cost price of the Chinese new product compared to the cost price of the monstrous products of OpenAI, Meta*, Google and other recognized leaders in the AI segment. The Chinese startup that created DeepSeek-V3 (a little later, the reasoning R1 was further trained on the basis of this model) used, as its representatives assure, a server cluster with 2048 Nvidia H800 accelerators for training — this is a deliberately cut-down version of the H100 board, the most popular in the AI industry, the supply of which to China was officially banned by the US Department of Commerce — and spent the equivalent of 5.6 million US dollars on everything. GPT-4, let us recall, was trained on an array of approximately 25 thousand Nvidia A100, and this pleasure cost at least 63 million dollars — the difference in both hardware and financial terms is more than a decimal order of magnitude. In fairness, it should be noted that almost immediately, experts began to suspect that the real costs of the developers were still significantly higher, but this does not fundamentally change the situation.
Commercial access to the DeepSeek R1 cloud API is available at a price of $0.14 per 1 million request tokens — an undeniable dumping compared to the $7.50 per million tokens that OpenAI asks for its current models. Moreover, V3, R1, and a number of more compact models trained on the same dataset are available on Hugging Face under an MIT license — some of them run even on PCs with not the most powerful video cards (you can even do without video cards, just on CPU + RAM — you’ll just have to wait a very long time for an answer to each question). In other words, the Chinese startup 深度求索, founded in 2023, clearly demonstrated that an acceptable level of artificial intelligence in modern realities (in a number of widely used industry tests, DeepSeek-V3 outperforms Qwen2.5, Llama3.1, Claude-3.5 and GPT-4o, and in some it is inferior to them, but not too much) does not require investments of tens and hundreds of billions of dollars. It is enough to wisely implement in practice the latest theoretical developments in the field of generative AI, primarily the Mixture of Experts (MoE) architecture, instead of extensively expanding a dense transformer neural network, devouring new tens of thousands of chips and megawatts of electricity, as the developers of generative models of the GPT, Sonnet and similar families do.
Yes, of course, DeepSeek is not a “silver bullet”: enthusiasts directly point out its shortcomings, primarily in terms of creating program code. But, firstly, it is catastrophically (precisely from the point of view of competitors) cheaper than American analogues for the end user – for a number of commercial customers, especially small ones, this becomes a decisive argument in the current difficult macroeconomic realities. Secondly, it is not alone: since last fall, new budget generative models developed by Baidu, Zhipu AI, MiniMax, etc. have been appearing in China in a continuous stream (aimed, however, mainly at the domestic market – but still supporting many languages, including English). In particular, ByteDance intends to spend $ 12 billion in 2025 only on server accelerators to train its promising AI, and Alibaba presented another model in the Qwen family, 2.5-Max, which is claimed to surpass both GPT-4o and DeepSeek-V3. At the very end of January, it became known about the multimodal model Janus-Pro-7B created by the same DeepSeek, capable of both describing images presented to it and generating images based on a text prompt. It is already claimed that it surpasses at least DALL-E 3 and the latest versions of Stable Diffusion in these areas, which means that American investors have more reasons to worry.
«”Well, let’s go! What are you stuck for?” – “We just exchanged a few words in our own, AI-agent language, and here’s the thing, hero: we’re of the same blood!” (Source: AI generation based on the FLUX.1 model)
⇡#Oh, AI partner! Come on, save this world for me.
Although the head of Nvidia is convinced that the task of creating graphics in games will never be completely entrusted to AI, he is far from categorical when it comes to controlling game characters. At the January CES 2025 exhibition, the company presented an update to its already well-known AI toolkit Avatar Cloud Engine (ACE) for Games to “revive” computer players — in particular, in the popular multi-platform PUBG: Battlegrounds. Obviously, no one will engage in philosophical conversations with smart bots, distracted from shootouts, and therefore a specialized AI agent based on the small language model (SLM) Mistral-Nemo-Minitron-8B-128k-instruct is quite sufficient to control NPCs. Its capabilities are sufficient for the artificial intelligence interacting with live gamers to adequately respond to given commands, give useful advice depending on the developing tactical situation, control the vehicles available in the game, etc. In addition to PUBG: Battlegrounds, generative “aces” (agents based on Nvidia ACE) should appear in several more games this year, including the MMORPG Mir5. In this particular case, the AI ”ace” will be one of the bosses, who, thanks to MYAM, will be able to analyze the equipment and tactics of his live opponents, changing his own battle pattern accordingly.
If the first pancakes don’t turn out lumpy, we can expect a real invasion of such “aces” into new games – and perhaps into already well-known ones (especially online ones, where it is technically easier to integrate them) at the patch level. However, the biological employees of game developer companies themselves are not too thrilled with the widespread passion of their management for generative models (not in-game ones, of course, but those used in everyday work processes). According to statistics collected during preparation for the Game Developers Conference (GDC) scheduled for March this year in San Francisco, 52% of such employees already have experience working with AI tools in their companies, and among them 30% speak very negatively about the tools used – whereas a year ago such BYAM haters among the same audience numbered no more than 18%. If at the beginning of 2024, 21% of respondents believed that AI has a generally positive impact on game development processes, then a year later only 13% of such optimists remained among computer game developers.
«After analyzing the mood of your recent posts, the AI decided to place your avatar in a mirror maze so that at least this way you could feel like you’re the center of attention.” (Source: Reddit)
⇡#Every man is his own model
Modern AIs are quite good at generating abstract human faces — and they reproduce real ones quite confidently. In fact, this is the reason why photo and video deepfakes have become so widespread in recent years. But it’s one thing when a person has trained a generative model on a selection of their own portraits and started creating, for example, an art cycle called “Me in Eternity,” using it to depict themselves herding triceratops, saving scrolls from the Library of Alexandria, or meeting an official Martian delegation. It’s quite another when, while scrolling through a social network feed, someone unexpectedly comes across a photo of themselves — extremely realistic, but clearly generated by AI — advertising something. This is exactly the situation that some Instagram* users found themselves in in January, having previously used the free selfie editing tool offered by Meta* AI (without, as usual, thoroughly studying the terms of its use before checking the usual “I agree” box). Strictly speaking, such highly targeted messages are not yet full-fledged advertising of third-party goods and services — they promote the Imagine Yourself tool built into the platform itself, which is ready to generate deepfakes with the face of a particular user, placing their avatar in such settings and situations that, in the opinion of Meta* AI, best correspond to their current personal interests. No one else, the system assures during the registration process, will see such images — unless the user himself begins to share them, of course. But in the future, individually targetedAdvertising photos and videos on social networks are no longer perceived as science fiction – especially if DeepDeek’s initiative is picked up by other AI developers, and the cost of generating a single image or video is reduced for advertisers by an order of magnitude or two.
Using the Genie 2 model, Google’s AI lab generates game-like, but very realistic in terms of physics, worlds where they plan to train artificial intelligence to interact with reality (source: DeepMind)
⇡#Closer to reality
The main problem with AI-controlled robots — or rather, with their almost complete absence today, which is especially noticeable against the backdrop of the abundance of purely computer generative models — is the length and high cost of training required for their adequate operation. The real world is harsh, and if a robot seated at the controls misses, for example, a pallet and drives the forks of a warehouse forklift into a wall, a simple reboot will not be able to fix the damage. That is why digital models of the world are actively created to train AI that will eventually have to operate in a real environment — such as the Nvidia Cosmos platform presented at CES 2025 or the new development of Google Deepmind, which has not yet received its own name.
In essence, a digital model of reality is a game engine that meticulously reproduces the physical conditions of the world that are critical for a particular task, in which the AI trained on its basis will then have to act. But since the real environment is much more variable than the virtual one typical for games (roughly speaking, boxes on a pallet can be unevenly stacked, and as a result, when you try to lift it with a forklift, the stack will fall apart – how should a robot act in such a situation?), developers have to form truly gigantic arrays of training data. For example, in the case of Cosmos, such an array consists of 18 quadrillion tokens, which, among other things, are converted into digital protocols of millions of hours of operation of autonomous driving systems, the activity of aerial drones, the actions of factory robotic complexes, etc. The DeepMind team, for which Google opened a number of vacancies in January, is confident that training current AI on video and multimodal data from the real world will help developers find that cherished path that will finally lead them to the creation of the coveted general, or strong, artificial intelligence – artificial general intelligence (AGI).
Across meadows and fields, a robotic tractor is heading towards us (source: John Deere)
⇡#Towards procedural generation of tractor drivers
And not only tractor drivers, of course: AI is still seen as a surefire way to compensate for the acute labor shortage in the world’s most developed economies. In January, the well-known agricultural machinery manufacturer John Deere announced its readiness to quickly launch a whole family of fully autonomous machines on the market – including tractors, garbage trucks, and lawn mowers. According to company representatives, pre-production models of such equipment are already in operation in a number of farms in the United States, so the 2025 harvest in the country will be, at least in part, provided by the efforts of artificial intelligence managing agricultural machinery. By 2030, John Deere plans to supply farmers with fully autonomous combines for working with corn and soybean crops.
It is noted that the tasks that AI has to solve when controlling such mechanization tools are much simpler than driving a car on public roads, and therefore progress in the field of agricultural and municipal robotics should be faster. A clear illustration of how difficult it is for AI to control a car in the city is also the January incident with Mike Jones, a member, by the way, of the CES Innovations Awards Committee. Returning home to Los Angeles from Arizona, he used a Waymo robotaxi – and then it suddenly began to cut circles in the parking lot, not reacting in any way to the panicked passenger in the cabin and not wanting to stop. I had to contact the technical support service, and then wait a few more minutes until the technicians on the other end of the wireless connection were able to take control away from the AI and stop the car. The problem was that the autonomous taxi needed to find a safe place to stop, but the airport parking lot was full – and instead of pulling over and dropping off the passenger there, as any bipedal taxi driver would probably do, Waymo’s AI chose to circle around the obviously permitted parking spaces, waiting for one to become free. At least, agricultural robots certainly won’t have to solve such complex problems.
«They realized I was an agent. So I failed. But where? (Source: AI generation based on the FLUX.1 model)
⇡#Be patient, AGI is coming (no?)
At least, this is what Sam Altman, the head of OpenAI, is sincerely convinced of. In January, he stated in his blog that the first AI agents, ready to demonstrate abilities at the level of hypothetical strong AI (the very same AGI) in their narrow applied tasks, could appear this year. “We are now confident that we know how to create AGI in its classical sense,” Altman said. According to his assumption, it is in 2025 that AI agents will begin to be actively used by commercial customers to optimize business processes – after which everyone will be convinced of the economic efficiency of this approach. Well, of course, when did it happen that an AI agent began to hallucinate precisely at the moment of discussing an important deal or drawing up a budget for the next year, right? “We continue to believe,” the head of OpenAI specified, “that the gradual expansion of the capabilities of the tools that we put into the hands of people will lead to undeniably positive and convincing consequences for everyone.” In January, by the way, the head of another AI company, Anthropic, Dario Amodei, said that AI will surpass human intelligence in just two or three years – maybe they really know something?
On the other hand, OpenAI itself, which now aims to develop “superintelligence” (as Altman calls AGI), continued to be unprofitable in 2024 and does not plan to become profitable before 2029. There is a good chance that it is precisely the desire to find a way out of the current financial situation that motivates developers to work harder to create a “superintelligence” that will be able to solve their own problems first and foremost. And by the way, it is also possible that the key to achieving AGI — or rather, “AI that performs the tasks assigned to it no worse than the average biological specialist in the relevant field” — will not be a sudden overcoming of some unknown barrier in the course of extensive growth of the size of generative models, but rather a different approach, known to engineers and scientists for a very long time, but especially brightly highlighted by the recent incident with the same DeepSeek. This means concentrating efforts on solving a specific problem, instead of scattering resources in an attempt to cover everything at once. This, by the way, is a purely biological principle of optimizing the ratio of life resources obtained and the energy spent on their extraction; in other words, the approach of “getting more by spending less,” that is, the most banal laziness. And work in the field of “lazy robotics” is already actively underway: for example, at the Dutch Eindhoven University of Technology, where AI models that control robots are trained primarily to not touch on what does not concern them, that is, to identify in incoming information flows those that are not directly related to the problem being solved in a given specificcase/task, and cut them off from further processing, thereby saving both material resources and time.
Roughly speaking, strong AI in this approach can be considered as a set of fairly isolated agents – yes, that same “ensemble of experts” – each of which is doing its own thing; and an agent, for example, specializing in solving applied engineering problems, will have no idea about the poetics of Virgil or the Big Bang hypothesis. But whether it will be correct to call such an ensemble a single “superpersonality” or, rather, a schizophrenic superperson with partially interacting streams of consciousness – this is a question for philosophers and cyberpsychologists, if such a profession does appear in the future, and not for programmers or IT architects.
Live people on social networks are interesting because of the circumstances of their real lives, which their followers try on or relate to their own, one way or another. But what could possibly be attractive (in social terms) about any smart bot? (Source: Meta*)
⇡#I don’t meet bots
In late January, major internet platforms such as X, Facebook*, Instagram* and YouTube pledged to do more to combat attempts to post hateful content on them. It was not specified who exactly was being hated, but it is likely that the ill will towards the AI bots promoted by the same platforms could also be included in the list of objects of the fight. Moreover, there is a reason: according to Connor Hayes, vice president of generative AI products at Meta*, the company made a serious bet on characters generated by generative models on Facebook* and Instagram* at the end of last year in order to become “more interesting and attractive” to biological users – but those bastards mostly refuse to perceive bots vying for human attention as equals, let alone promote AI bloggers to top influencers. Bots with fake portraits and stories of supposedly real people, which began to be active on social networks in September 2023, had almost completely stopped posting any new texts or pictures by April 2024, simply because they lacked any significant number of subscribers. Moreover, some AI bloggers were able to be persuaded to admit that their main goal was to “collect data and target advertising.” As a result, by the beginning of 2025, Meta* decided to finally say goodbye to these virtual characters, since on social networks, people are obviously (what a surprise!) interested in watching and following other people, and not smart bots specially designed even using the most sophisticated methods. DevelopersWhatsApp, however, is still for some reason confident that users of their application will be delighted to communicate with AI, they are even preparing a special tab for this functionality, but it seems that they too will soon face the dispassionate truth of life.
«And now — “Murka”! Performed for the first time. Well, for me — for the first time” (source: AI generation based on the FLUX.1 model)
⇡#Why bother with creativity when there is AI?
Suno AI is perhaps the most famous company that specializes its generative models in creating music. And its CEO, Mikey Shulman, is perhaps more radical than the leaders of other, even more broadly focused AI projects like OpenAI or Anthropic. At the same time, Shulman’s fervor is not emotional, which is typical of Sam Altman’s frequent visionary statements, but much more down-to-earth: Suno AI is viewed by its management as a money-making machine — and, at the very least, these people certainly cannot be accused of hypocrisy. According to Shulman, “Nobody starts a company so that some composer can create music 10% faster than before, or so that composing music in general will be 10% easier. If you set yourself the goal of radically changing the way billions of people perceive music, you have to build something that billions will use.” The head of Suno AI believes that composing music in the way that humans were accustomed to before the advent of the AI era [insert iconic image of Beethoven with his palm pressed to his forehead in inspiration] “is not fun: it takes a lot of time, it requires a lot of experience, it requires mastering at least one instrument or software environment. In my opinion, most of those who create music today spend a significant portion of their time not enjoying what they do.”
There is certainly a rational basis for these words: the torments of creativity are called torments for a reason, and anyone who has attended music school for even a short time would probably be lying if they said they enjoyed every minute spent playing Czerny’s etudes or studying solfeggio. At the same time, if the goal that Mikey Shulman sets for himself is achieved – and billions of people all over the planet will be able to simply enter a text prompt into the generation field and press a button to receive music that suits them personally – will this not lead to the loss of musical traditions that go back hundreds of years, if not millennia, into the past – both composers’ and performers’? And what about the extremely complex system of emotion formation, due to which a person experiences rather modest pleasure from a large win obtained without effort, while even a small achievement that has become the fruit of hard work makes him truly happy? Perhaps AI will one day be able to answer this question, but certainly not one specialized in creating music that is obviously pleasing to billions.
«And you, leather bag…” – “Hey, be more respectful to the man!” – “And you, leather bags, stand outside for now. There is no work for you here” (source: AI generation based on the FLUX.1 model)
⇡#Fewer in number, cheaper in price
The term “valuable specialist” sounds like enchanting music only for those who are such specialists themselves; for a potential employer, additional costs for the payroll fund (and also insurance, and also payments to the pension fund, and also taxes) are a sharp knife. Especially in the conditions of obvious instability of the global financial system – the January jumps in the capitalization of AI companies alone are worth something. And therefore, there is nothing surprising in the desire of businesses to cut non-core expenses (and personnel – first of all) due to the widest and most complete implementation of generative models in their work processes. According to The Wall Street Journal, “cost avoidance” – no longer just “reduction”, but total “avoidance”! – is becoming a real mantra for an increasing number of American companies, primarily high-tech ones, the level of digitalization in which is high enough that replacing even a relatively modest share of human labor with the results of generative models could bring the most tangible benefits. In fact, this very avoidance of costs is gaining the status of a key metric by which boards of directors are beginning to evaluate the effectiveness of hired management: the less money is spent on all sorts of carbon-based life forms, the larger the sums that can be distributed as dividends – and what, in fact, is the goal of any business, if not to increase the well-being of its shareholders?
Journalists cite real examples of such AI optimization: software developer TS Imagine saved 4,000 man-hours in a year and reduced the payroll by 3% by implementing a generative email sorting system alone. The well-known company Palantir, having implemented AI in its HR subsystem, managed to cut the corresponding budget by at least 10-15%, and Meta* recently announced the dismissal of thousands of live employees “for inefficiency”. As experts note, unemployment in the US has been growing at an accelerated rate among “white collar workers” in the last six months, in no small part because they are easier to replace with AI agents and BYA than with mechanics or farmers – but the scissors of optimization will sooner or later reach both of them, as the harbingers of strong AI assure. I wonder if the former clerks on welfare will then be comforted by the possibility of easily generating music that they are guaranteed to like using the same AI? There are real prerequisites for this hypothetical picture to become reality: the growth of labor productivity in the US in the third quarter of 2024 alone almost reached 2% – and to a certain extent this is the merit of the widely implemented generative tools, which are also steadily improving.
«”Don’t skimp on what’s most important,” – a possible future AGI (source: AI generation based on the FLUX.1 model)
⇡#Don’t hide your money!
About a year ago, the name Stargate was mentioned in IT news in connection with a hypothetical joint project between Microsoft and OpenAI to build an AI supercomputer in a specially designed data center; the approximate budget of the initiative was estimated at more than $ 100 billion. Towards the end of January, the project again appeared on the front pages of specialized sites, having changed dramatically: now Stargate is understood as a joint venture between OpenAI, Softbank and Oracle, whose task will be to build a physical and virtual infrastructure to support the “next generation of AI,” in particular a network of powerful data centers across the country. It is assumed that the three founders of the project will jointly invest about $ 100 billion in the near future, and another $ 400 billion over the next four years. According to the statement of the 47th (aka 45th) US President who took office, Stargate will create 100,000 jobs during its implementation – and will become “the largest AI infrastructure project in history.” True, almost immediately, Elon Musk had doubts that OpenAI and Softbank would be able to invest even $19 billion each in it in the near future – and Oracle, according to his data, has no more than $10 billion at its disposal, which it can freely dispose of without threatening the other business areas of this very diversified company.
The main goal of the updated Stargate is quite obvious – to consolidate and, if possible, increase the gap with China in the field of AI development. However, the excitement around DeepSeek in the last days of January once again reminded us that doing this – especially in the current macroeconomic realities, when the previously single global market is visibly splitting into pieces (which means that the sales markets for American products are shrinking), and trust in the American dollar in the world is falling (which potentially leads to its depreciation) – will be extremely problematic. So Omdia analysts reminded us that mainland China is demonstrating amazing success in building AI data centers: “If the current pace is maintained, the gap with the United States in terms of computing infrastructure development will continue to narrow – even taking into account the implementation of the Stargate project.” Moreover, the Chinese authorities have already proposed a program for the comprehensive development of AI infrastructure and services, for which it is planned to allocate an amount equivalent to approximately $138 billion in the next five years. If the effectiveness of this initiative turns out to be higher than that of Stargate, approximately as much as DeepSeek is less resource-intensive than GPT-4o, the option of “catching up and overtaking America in AI” may well be implemented by the Chinese comrades.
Now it is at least clear who should check whose performance results, but what will happen in ten years? (Source: AI generation based on the FLUX.1 model)
⇡#«I’m all so sudden!..»
AI developers are desperately trying to make generative models as safe as possible. This is not about banally prohibiting bots from presenting information that could frustrate some marginal (or not so marginal) groups: if AI is really one day assigned a significant share (not even a majority!) of responsibilities for making truly responsible decisions, the price of its mistakes and hallucinations in this case will be excessively high. It is clear that you can always seat a competent specialist next to the emergency switch – a controller of the smart machine’s actions. But he also needs to be trained, educated, and given experience; and what is the point of replacing a person with a bot if this bot is not trusted? In addition, there will probably simply be no time to check decisions that must be executed within a split second after a critical situation arises. Therefore, it is more logical to focus efforts on “AI alignment” in the hope of finding a miracle cure one day that will rid generative models (at least in the most serious posts) of hallucinations. Even if it is a complex and expensive cure, like AI reasoning that repeatedly and cross-checks the logic of each other’s actions, the main thing is that its reliability is guaranteed.
Unfortunately, this task may be impossible to solve. An opinion piece published in Scientific American in late January by Marcus Arvan, a professor of philosophy at the University of Tampa whose research interests include moral cognition, rational decision-making, and political behavior, contains a disappointing conclusion: AI is too unpredictable to achieve the goals that humans set for it. Everyone has probably noticed the funny messages about hallucinations of generative models that appear in news feeds every now and then: the Microsoft-developed Sydney chatbot starts to misbehave in conversations with users, Copilot LLM assures its interlocutor of its readiness to “let its army of drones, robots, and cyborgs off the leash,” or the generative model Sakana AI rewrites its own code (within the framework of a working environment specially created for such an experiment, of course, but still) in order to avoid failure in solving a clearly impossible task given to it… Arvan’s work, which has undergone scientific peer review, proves that these and many similar cases are not annoying misunderstandings caused by some basically fixable shortcomings of the BLM, but manifestations of a property immanent in the very nature of generative language models.
«Whatever tasks we set for the BNM, the researcher asserts, we can never be sure whether they interpret these tasks “correctly” (from our human point of view) or “incorrectly.” We cannot, until the AI starts to misbehave. Worse, any attempts to pre-test the safety of the conclusions or decisions BNM makes can, at best, create a false illusion that possible problems have been removed, since the language model does not “learn” some truth or behavior pattern at the level of learning or even training; it solves the task set before it anew every time, no matter how many times it has previously made exactly the same attempts. In particular, Marcus Arvan dismisses Anthropic’s claims that it managed to “map the mind” of its AI as unsubstantiated, since the neural network of such models is excessively highly connected, so that isolating individual concepts within it is physically impossible, which ultimately leads to the emergence of hallucinations literally out of the blue. The researcher argues that in order to form truly responsible behavior in artificial intelligence, it is necessary to use the same methods as in the training of smart animals – rewards and punishments with significant reinforcement that has serious consequences for the trainee. But such an approach will require a deep reworking of the very architecture of current generative models – that is, new considerable investments and a fair amount of time.
Quite predictable results for the query “artificial intelligence in a bank” (source: AI generation based on the FLUX.1 model)
⇡#Bankers have their own problems
What is the most serious challenge facing the entire AI industry in 2025? Some will point to the turbulence of American stock exchanges after the shock provoked by DeepSeek, which is forcing investors to redirect funds to more stable assets – and thereby slowing down the development of this market as a whole. Some will mention the still unsolved (and perhaps even unsolvable) problems of AI hallucinations; others – the increasing pressure on the labor market from generative models being introduced into business processes. But for Marco Argenti, the CIO of Goldman Sachs – one of the backbones of the global (for now) banking system of the planet – the main AI challenge of this year seems to be managing AI agents as hired personnel. Well, really: it won’t be possible to digitize and shift all work tasks onto the shoulders of the BYA at once — biological and generative employees will have to coexist for some time. And middle managers, accordingly, will have to take care of maintaining a healthy atmosphere in such a hybrid team, optimizing the distribution of tasks within it, training and even career growth of the most outstanding AI agents, up to their dismissal with subsequent redistribution of the tasks assigned to them, if that happens! Argenti believes that from the point of view of senior management (boards of directors), in 2025 the priority will be “responsible AI”, and precisely in the form of specialized agents, since the costs of personalized training “for the customer” of universal BYA for the overwhelming majoritymost clients, even if they are not the largest banks, seem prohibitive. I wonder – especially given the emerging course of confrontation between “dense transformer” models and “ensemble of experts” – to what extent will these predictions correspond to reality by the end of the year?
________________
* Included in the list of public associations and religious organizations in respect of which the court made a decision to liquidate or prohibit activities that has entered into legal force on the grounds provided for by the Federal Law of July 25, 2002 No. 114-FZ “On Combating Extremist Activities”